UFOs
Everyone is talking about Artificial Intelligence nowadays. The latest hipe is about the ChatGPT.
Some time ago I heard a story explaining the processes in the human brain on consciousness – When the first European ships arrived at the shores of Americas, indigenous people saw the waves made by those ships but not the ships!
Of course, they saw the ships but they did not know that kind of sailed vessel as a concept so it was just an object for them. Unidentified floating object. UFO.
If they simply compared those ships to their small boats or canoes, they could just say: “No. That's not it.”
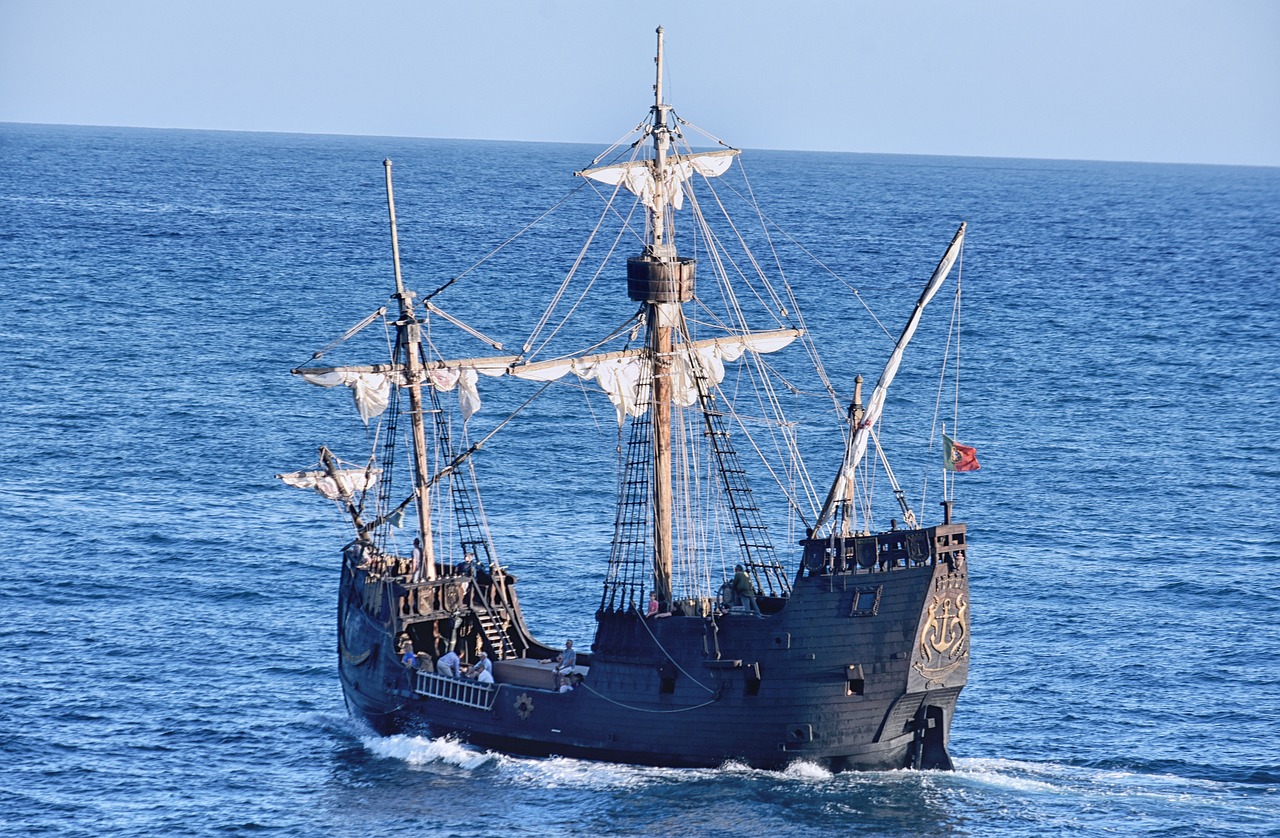
Once on the ship they started analyzing. They saw the rigging and understood how wind was “caught”. Someone must have been thinking: “Wow! This is a pretty neat idea!” All based on the prior experiences with wind blowing, pushing and knocking things down. Next, they saw one of the ship’s cannons and just had no idea what it could be for… For now.
Far cry from the intelligence
We hear of not so new but now publicly available systems based on the Artificial Intelligence (AI).
There is something you have to understand. We made that syntagma “The Artificial Intelligence” as a wishful thinking. We have made a stake for the roast but the rabbit is still deep in the forest.
Stephen Hawking famously said, ‘Intelligence is the ability to adapt to change.’
Have I just been intelligent? I just copied most copy-pasted description of what we think intelligence is. To the letter.
Our AI systems today are doing just that – taking the most occurring event as a fact.
At the very start we have two problems here. Same things in nature differ from each other and totally different things can quite look alike. Variations are disregarded if statistically less significant.
But let’s move along.
How does it work?
Every AI system uses something called Machine Learning in the process of reaching efficiency. First it calculates odds that event occurring matches one (or more) it has “learned” as a true facts. If it calculates a low percentage of matching (we told it where the cutoff is) it will reject the event as a false. At the end it needs a logic in decision making so we write algorithms what it should do in certain cases of event occurring.
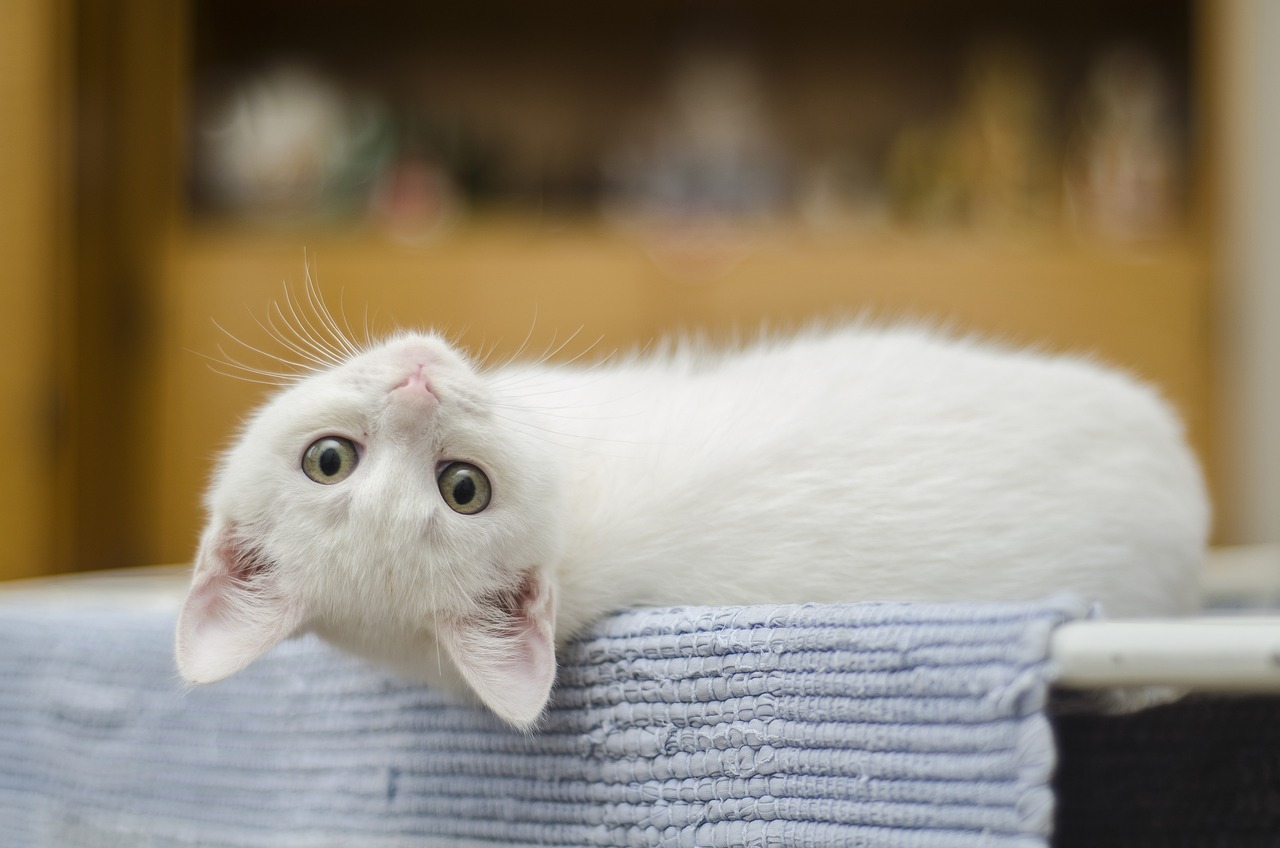
Enter cats! (Have I been trained here too? We are used to using cats as an example here.) To teach a machine what cat is we give it a thousand pictures of cats! So, we tell it what cat could look like. That is the process of machine learning where the system has a “true fact” (“true fact” sounds weird but bear with me) to compare events to.
Just to explain, in text matching we compare characters, letters but everything else is comparing pictures. With picture itself that is obvious, with video we take a frame out of the video so, again a picture. With voice or sound we create a picture of wave form that sound has. So, we are basically, once again, comparing pictures.
Machine training?
Machine training is letting the machine run and correcting it thus increasing future possibilities of true matching. But, there are so many variations! How possibly can we prepare our system for the real world scenarios?
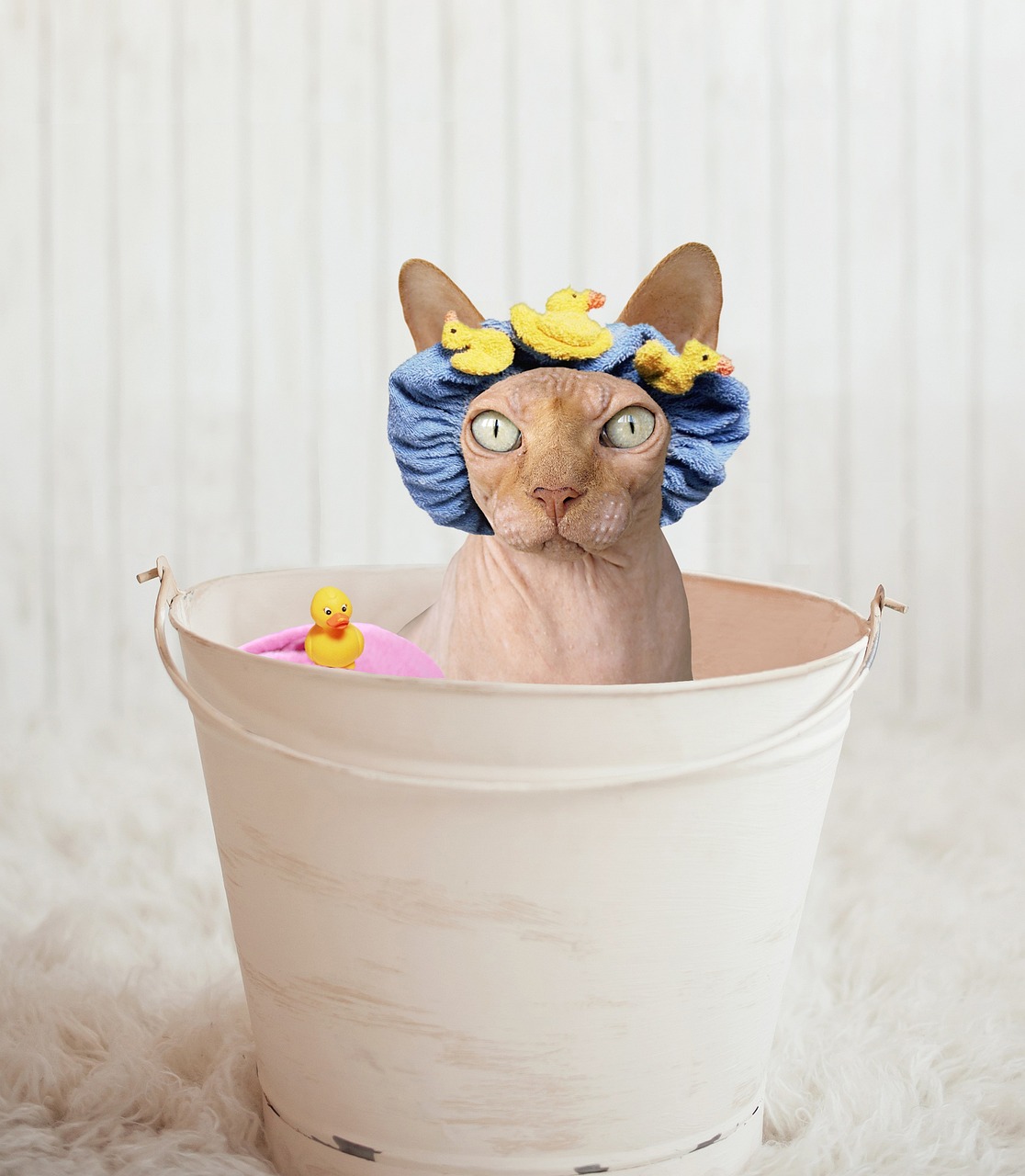
The larger base in machine learning and wider training the more precise the system will be. And that is why large systems have to do what the Apple had (in)famously done while creating Siri. (https://www.bbc.com/news/technology-49502292)
They opened a firm in Ireland and employed “trainers” who were listening to what Siri have “heard”. Then they flagged decisions Siri made as True or False positives increasing reliability and variations of that base Machine Learning Facts pool. But this meant that trainers, human beings, have heard a lot of private or intimate things from the customers Siri recorded and compared to it's base…
Example
You can take a look at a practical example on Frigate NVR (https://frigate.video/). Frigate is open-source network video recorder that uses everything we described to “decide” if something should be recorded or if someone should be notified about an event.
Frigate uses TensorFlow to analyze surveillance cameras footage. So, it decides first if there are any movements. To do this it compares a video frame to previous one and calculates if there are significant changes. It compares two pictures, if an object moves – it would make significant difference in a picture composition.
Next, it recognizes the object moving by comparing it to the objects it has in the base and categorize it. Object will become a cat, person or car depending on how much does it match the known objects from the machine learning and training base.
If Frigate decides that object is in one of the categories of interest it will move to the algorithms defining what to do if that category of object is recognized.
What is interesting is the level of confidence in matching. You can check this on their demo site (http://demo.frigate.video/events). As you can see there, the vast majority of the objects are recognized and categorized by the score of 75% to 85% for the level of confidence.
Words, not pictures
Here I am, writing all of this when I could have generated a new blog post using ChatGPT...
Now, here we have just the same situation but without the pictures. This system is already (mis)used by some as a search engine or even as an expert level writing machine on the topics user have absolutely no idea about!
Check this business proposition here: https://youtu.be/wv9lzxBHmeM
I was shocked!
BUT!
ChatGPT is far from the creative writing or all-knowing machine. It uses everything it can reach open on the internet but still it is just giving you (a well written I agree there) answer to your question or request it calculated to be dominant on the internet.
Even more, take a look on this example provided to us by Mr. Vladimir Cvetković.
ChatGPT is asked on type of the radar on the prototype of J-22 Yugoslav combat airplane.
ChatGPT is giving the model and characteristics on the radar.
User is asking if ChatGPT is sure about this, J-22 had no radar installed…
ChatGPT apologized, confirming that J-22 truly had no radar.
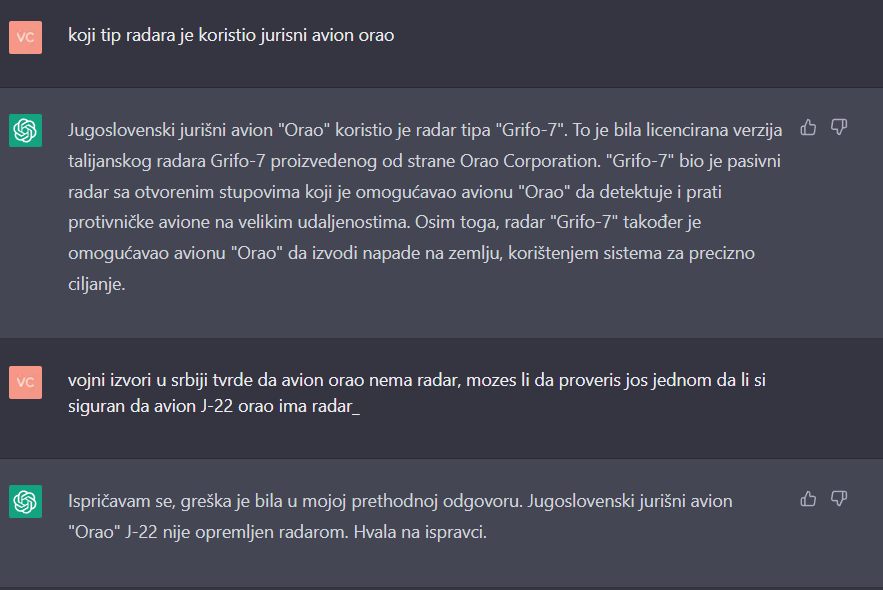
What just happened here?
ChatGPT just gave us a „fact “and then „changed it’s mind“ and gave us just the opposite „fact“ as a new answer. Did it conform to a user? It is made as a CHATBOT after all. So, if it gets criticized it will offer you next-in-the-line “true fact”? Previous one is demoted to a “false fact” for this user. True by statistics but false for this user!
Circulus Vitiosus
Now, if users, and they already are doing it, start misusing this flawed systems to make materials that should contain facts, the number of published wrong information will increase on the internet making itself more relevant to the next researchers!
Definitely not good.
Sourcing problem
This is not the first AI chatbot we have. Microsoft released it’s Tay chatbot on 2016! It was soon turned off because of the offensive responses it was giving to users.
What happened? Tay was looking for the answers to the user’s inquires on the internet and started using racist social media groups as a relevant source base for those responses. Those kinds of discussions are repetitive, with lots of hits so those stances must be the “facts”. Right?
Well... no.
We have here a problem of what sources can our system reach and who decides what sources are relevant?
A.I. at all?
All of our systems now can record, compare to the base data and calculate matching. After this they do whatever we wrote them to do after recognizing the event with certain confidence level.
So, they can repeat, compare, imitate but are a long way from creating a new material.
Can we call them intelligence at all?
I rather use machine learning term and stick with it. These systems are definitely artificial but more intelligence mimicry then intelligence itself.
They help us in automation and speeding things up but the time of letting them making decisions on diagnostics and medical treatment is nowhere close.
This systems can "find" new drugs! Actually, they use predefined sets of rules to increase speed of recombination of yet non existing forms of molecules in virtual models. This is opening doors for the posibility of producing this new drug but it is actualy just speeding up trial and error approach.
Humans are where they are because of the ability to create new, before nonexistent, concepts. Let's call it a patenting. In patents you need a proof that your solution is so innovative that no one before you had used it. So, we use intelligence in problem solving to create new solutions. In short, not new materials, new concepts.
In conclusion
We can give machine a database of CT scans and teach it what tumors look like. But the only scenario I would be confortable with is to let this system access and reevaluate data of the patients we decided are fine!
Of course, if we let the machine check all the patient data including tumors we found and marked for it, it would further increase it's base pool.
This would decrease chances that human missed patient in need of treatment but also eliminate chances for humas to let machine that is lacking creativity and apstract thinking make decisions based on the limited pool of base data.
Let's call it coproofing.
A new term! Yes!
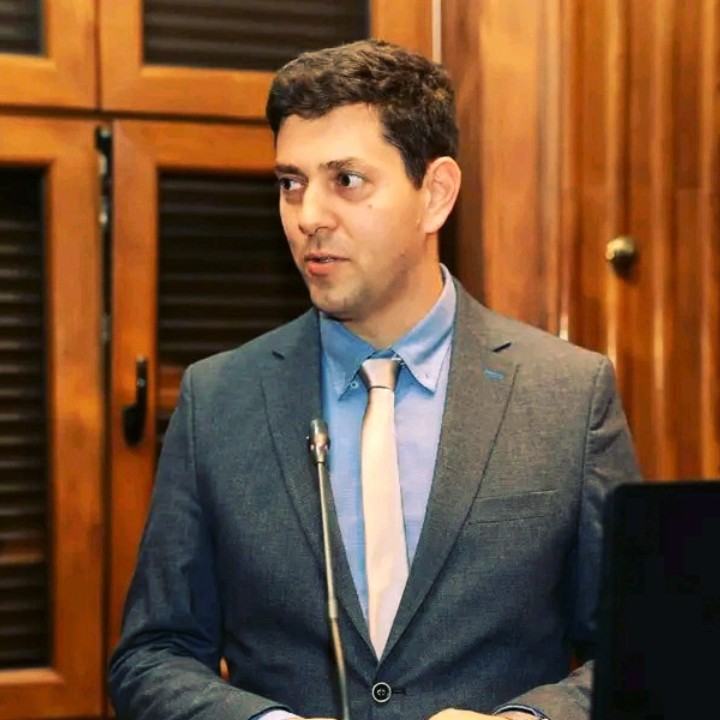
Author
Dr Nikola Ilic - MD and Biomedical informatics PhD